RAGChecker: Transforming AI Evaluation for Accuracy
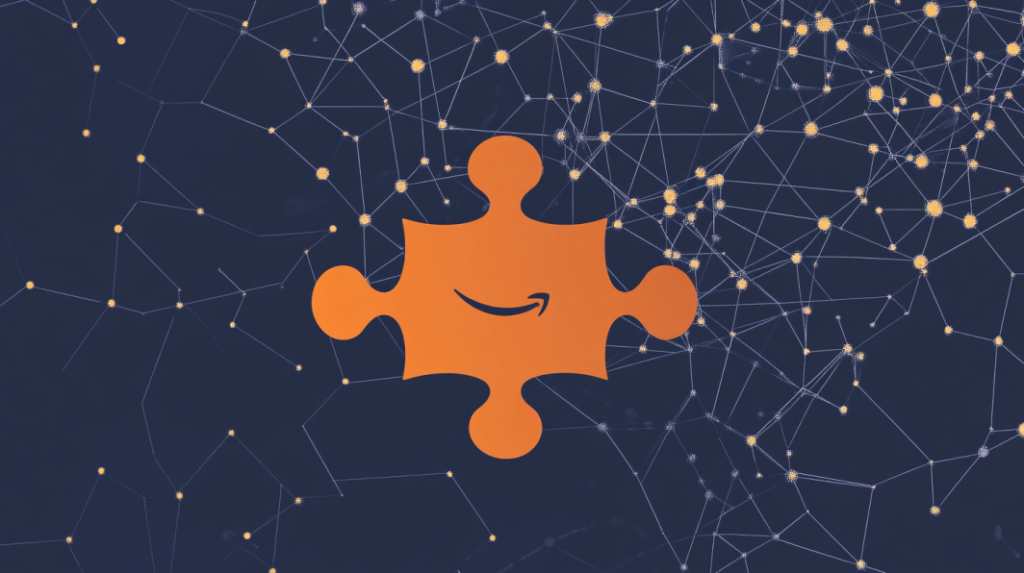
RAGChecker: A New Dawn in AI Evaluation
In the rapidly advancing world of artificial intelligence, the quest for accuracy and relevance in AI responses has never been more critical. As organizations increasingly rely on AI for tasks demanding up-to-date and factual information—such as legal advice, medical diagnosis, and complex financial analysis—the tools used to evaluate these systems must evolve. The introduction of RAGChecker by the AWS AI team marks a pivotal moment in this journey, offering a sophisticated framework designed to enhance the evaluation of Retrieval Augmented Generation (RAG) systems.
Understanding RAG Systems
RAG systems are at the forefront of AI technology, combining large language models with external databases to generate contextually relevant answers. However, existing evaluation methods often fail to address the intricate challenges that arise within these systems. RAGChecker addresses this gap by providing a more nuanced approach to evaluation, focusing on:
- Claim Level Entailment: This method allows for a detailed analysis of the retrieval and generation components of RAG systems.
- Holistic Performance Metrics: By offering overall metrics, RAGChecker enables enterprises to compare different RAG systems effectively.
Dual Purpose Tool
RAGChecker is not merely a tool for researchers; it holds significant implications for enterprises as well. It provides:
- Overall Metrics: Giving companies a broad view of system performance.
- Diagnostic Metrics: Highlighting specific weaknesses in retrieval or generation phases, thus allowing for targeted improvements.
Types of Errors Addressed
The tool identifies two primary types of errors that can occur in RAG systems:
- Retrieval Errors: Instances where the system fails to find the most relevant information.
- Generator Errors: Situations where the system struggles to utilize the retrieved information accurately.
By distinguishing these errors, RAGChecker can guide developers in diagnosing and correcting issues, leading to enhanced system performance.
Testing Across Critical Domains
Amazon’s team has rigorously tested RAGChecker on eight different RAG systems using a benchmark dataset that spans distinct domains, including medicine, finance, and law. Key findings from this testing include:
- Trade-offs in Performance: Systems excelling at retrieval often bring in irrelevant data, complicating the generation phase.
- Faithfulness of Generators: Once relevant information is retrieved, systems may overly rely on it, even if that information contains errors or misleading content.
Interestingly, differences between open-source and proprietary models have also been observed. Open-source models tend to trust the context provided more blindly, leading to potential inaccuracies in their responses.
Implications for High-Stakes Applications
For businesses that depend on AI-generated content, RAGChecker could prove invaluable. By offering a detailed evaluation of how these systems retrieve and utilize information, it ensures that AI remains accurate and reliable, particularly in high-stakes environments.
As artificial intelligence continues to evolve, tools like RAGChecker will be essential in striking a balance between innovation and reliability. The metrics offered by RAGChecker promise to guide researchers and practitioners in developing more effective RAG systems, potentially transforming the landscape of AI applications across various industries.
The anticipation surrounding RAGChecker’s eventual public release underscores its potential impact, not only for developers and researchers but for the broader industry landscape as well. As organizations await further announcements regarding its availability, the excitement for a new standard in AI evaluation grows.
Comments
Post a Comment